Li Fei-Fei's Team Trains AI Model for Under $50, Revolutionizing Industry Standards
The breakthrough innovation by Li Fei-Fei's team, which successfully trained a new model, S1, with a cloud computing cost of less than $50, has sparked a reevaluation of the development costs associated with artificial intelligence. This achievement is remarkable, given that S1's performance in mathematical and coding ability tests is comparable to that of top-tier models like OpenAI's O1 and DeepSeek's R1. The research, conducted by Li Fei-Fei and her colleagues from Stanford University and the University of Washington, demonstrates that with careful selection of training data and the application of distillation techniques, it is possible to create highly competent AI models at a fraction of the cost typically associated with such endeavors.
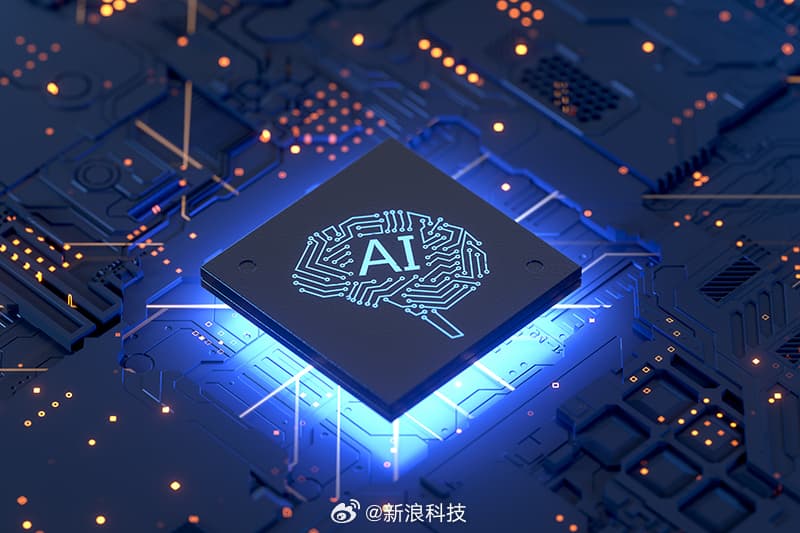
6 February 2025
The S1 model's data was derived from Alibaba Cloud's Qwen2.5 model through a process of distillation, utilizing a novel approach called test-time scaling. This method involves constructing a small, yet carefully curated dataset with 1000 selectively chosen questions and answers, including detailed reasoning processes. Training was completed in just 26 minutes using 16 NVIDIA H100 GPUs, highlighting the efficiency and cost-effectiveness of this approach compared to traditional large-scale reinforcement learning methods employed by DeepSeek and OpenAI. Furthermore, the researchers employed a "budget-forcing" technique to enhance the accuracy of the model's responses, allowing for the control of computation time during testing and optimizing performance.
The emergence of S1 has also raised concerns within the industry, particularly regarding the potential impact on the significant investments made by large AI companies in research and development. OpenAI has previously accused DeepSeek of improperly using its API data for distillation purposes, raising questions about the ethical and legal boundaries of model distillation. Meanwhile, analysts have expressed skepticism about whether the ease of replicating and surpassing existing top models could undermine the value of years of R&D and technological advancements by major AI players.
This groundbreaking achievement by Li Fei-Fei's team not only challenges the conventional wisdom on AI development costs but also opens up new avenues for research into efficient and cost-effective methods for training AI models. As the AI landscape continues to evolve, innovations like S1 are poised to play a pivotal role in making advanced AI capabilities more accessible and affordable, potentially democratizing access to AI technology across various sectors and applications. The use of model distillation techniques, as demonstrated by Li Fei-Fei's team, could become a focal point for future research and development, enabling entities to bypass the need for extensive, resource-intensive training processes.
However, this development also raises important questions regarding intellectual property, data privacy, and the potential for misuse of AI technologies. As models become more accessible and affordable, there will be a growing need for robust regulatory frameworks to ensure that these technologies are developed and deployed responsibly. The journey ahead will require careful navigation, collaboration, and a commitment to responsible AI development.
The achievement by Li Fei-Fei's team has sparked debates about the future of AI development, with some questioning whether computing power will become oversupplied and whether distillation technology can replace traditional development methods. If distillation technology can be used to train high-performance models at a fraction of the cost, it could potentially disrupt the AI industry, leading to a shift towards more efficient and cost-effective methods of AI development. However, some experts have expressed concerns about the potential consequences of this shift, including the impact on large AI companies' research and development investments and the potential for misuse of AI technologies.
As the AI industry continues to evolve, it will be interesting to see how distillation technology and other cost-effective methods of AI development will change the landscape. The future of AI development will be shaped by innovations like Li Fei-Fei's team's breakthrough, which is pushing the boundaries of what is possible in the field. Ultimately, the key to harnessing the potential of AI lies in balancing the pursuit of innovation with the imperative of ensuring that these technologies contribute positively to society, fostering a future where AI enhances human capabilities without exacerbating existing challenges.